How AI Learn Itself: Understanding the Process of Self-Learning
Published: March 14, 2025
Artificial Intelligence (AI) is rapidly transforming the world around us. From self-driving cars to personalized recommendations on streaming platforms, AI is becoming a powerful tool in many industries. But have you ever wondered how AI actually learns and improves over time?
In this blog, we’ll explore the intriguing question: “How does AI learn by itself?” We’ll break down the key processes involved in AI learning, such as machine learning and neural networks, and explain how AI continuously improves its performance. Whether it’s making decisions or adapting to new situations, understanding how AI learns will give you a clearer picture of its potential and limitations. Let’s dive into how AI becomes smarter on its own and what makes it such an essential part of modern technology.
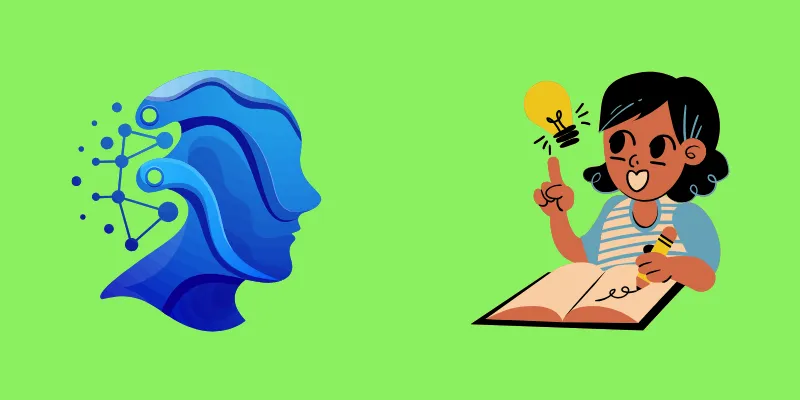
What is Machine Learning?
Definition of Machine Learning
Machine learning is a type of AI that allows computers to learn from data without being explicitly programmed. In simple terms, it’s like teaching a computer to recognize patterns and make decisions based on the information it receives. The more data the AI processes, the better it becomes at making predictions or solving problems.
Why AI Needs to Learn
For AI to be useful, it needs to learn from the data it receives in order to improve its performance over time. Without the ability to learn, AI would be limited to simple tasks and could not adapt to new information or make better decisions as time goes on. Learning allows AI to get smarter, adapt to new situations, and provide more accurate results, making it an essential tool in many industries.
Real-Life Example
A common example of machine learning in action is the recommendation system on platforms like Netflix. When you watch a show or movie, Netflix’s AI analyzes your viewing history and uses machine learning to recommend similar shows you might like. Over time, as you watch more content, the system learns your preferences and offers even more personalized suggestions based on what it has learned about your tastes.
How Does AI Learn?
Supervised Learning
Supervised learning is a type of machine learning where AI learns from labeled data. This means that the AI is given both input (data) and the correct output (label), and it learns to map the input to the correct output. For example, in email spam filters, AI is trained using a dataset of emails that are labeled as “spam” or “not spam.” By analyzing this labeled data, the AI learns to recognize patterns in the emails—such as certain words or phrases—and can then classify new emails into the correct categories (spam or not) automatically.
Unsupervised Learning
In unsupervised learning, AI works with data that does not have labels. Instead of being told the correct output, the AI tries to find patterns or groupings on its own. A great example is in online shopping platforms, where AI can group similar products based on their features (like price, color, or brand) without any predefined labels. For example, an AI system might group all red shoes or all running shoes together, helping users easily find similar items based on their preferences.
Reinforcement Learning
Reinforcement learning is a type of learning where AI learns through trial and error, similar to how humans or animals learn new skills. In this process, the AI takes actions in an environment and receives feedback in the form of rewards or penalties. Over time, it learns which actions lead to the best outcomes. A real-life example is a robot learning to walk. Initially, it may stumble and fall, but through reinforcement learning, the robot receives positive feedback when it takes a step in the right direction, and negative feedback when it falls. This helps the robot improve its walking skills over time.
Neural Networks: The Brain of AI
What Are Neural Networks?
Neural networks are a key part of how AI learns, and they’re inspired by the human brain. Just like our brain is made up of neurons that connect to each other, neural networks consist of layers of “artificial neurons” that are connected to each other. These neurons work together to process information, learn patterns, and make decisions. In simple terms, neural networks allow AI to understand and process complex data in a way that mimics how humans think and learn.
How Neural Networks Help AI Learn
Neural networks help AI learn by analyzing large amounts of data and recognizing patterns within it. The data passes through different layers of the network, where each layer helps the AI make sense of the information. The more data the network processes, the better it becomes at identifying patterns and making predictions. Over time, neural networks “learn” from this data and improve their ability to make accurate decisions or predictions.
Example
A real-world example of neural networks in action is facial recognition technology in smartphones. When you set up facial recognition, the AI uses a neural network to analyze the features of your face, like the distance between your eyes or the shape of your jaw. Once the AI processes enough data, it learns to recognize your face, allowing you to unlock your phone just by looking at it. This process relies heavily on neural networks to identify and remember patterns in the facial features.
How Does AI Improve Over Time?
Continuous Learning
One of the key ways AI improves over time is through continuous learning. As AI processes more data, it can refine its ability to make accurate predictions and decisions. The more examples it encounters, the better it becomes at recognizing patterns and making smarter choices. For instance, a recommendation system in a music app can learn to suggest songs that better match your taste as it gets more data about your listening habits.
Adaptation
AI also has the ability to adapt to new situations. This means that as AI encounters different or unfamiliar scenarios, it can adjust its behavior or response accordingly. For example, self-driving cars learn to handle various driving conditions—like rain, fog, or different types of road surfaces—by processing real-time data from their sensors and adjusting their driving strategies based on what they’ve learned. This allows AI to operate more effectively in ever-changing environments.
Example
A good example of AI improving over time is how streaming services, like Netflix or Spotify, refine their recommendations. As the AI gathers more data about your viewing or listening preferences, it becomes better at suggesting content you might enjoy. Initially, it might only recommend popular movies or songs, but as it learns more about your specific tastes, the recommendations become more personalized and accurate over time.
Benefits and Limitations of AI Learning
AI learning offers several significant advantages:
- Faster Decision-Making: AI can process and analyze data much faster than humans, making decisions in real-time and improving efficiency in many applications, like customer support or finance.
- Ability to Handle Large Amounts of Data: AI excels at processing huge volumes of data, something human brains cannot do quickly. This is especially useful in areas like healthcare, where AI can analyze medical records to detect patterns or predict health outcomes.
- Automation of Repetitive Tasks: AI can take over routine tasks, such as sorting emails or filling out forms, which frees up humans to focus on more complex and creative work.
Despite its advantages, AI learning has its limitations:
- Dependence on Data Quality: AI relies heavily on the quality of the data it is trained on. Poor or biased data can lead to inaccurate or flawed outcomes.
- Inability to Think Creatively: AI is limited to the patterns and information it has been trained on. It cannot come up with truly creative or innovative ideas, which humans excel at.
- Risk of Bias: If the data used to train AI is biased or incomplete, the AI can reflect these biases in its decision-making. This is a significant limitation in areas like hiring or lending, where AI might make unfair decisions based on biased training data.
Example
A common example of bias in AI is biased hiring algorithms. If an AI is trained on historical hiring data that reflects past biases (such as gender or racial bias), it can unintentionally make biased hiring decisions, favoring certain groups over others. This highlights the importance of ensuring that AI systems are trained on diverse and unbiased data.
Can AI Learn by Itself Without Human Input?
Current Capabilities
AI has made significant strides in learning and making decisions without direct human involvement. Through machine learning algorithms, AI can process vast amounts of data, recognize patterns, and make predictions or decisions based on what it has learned. In certain tasks, such as recommendation systems or customer service chatbots, AI can function with minimal human interaction once it is properly set up and trained. However, AI still needs initial guidance, like providing labeled data or setting up the system for learning, to begin its process.
The Role of Human Oversight
Despite its ability to learn independently, AI still requires human oversight to ensure it functions properly and ethically. Humans must guide the AI in the beginning and intervene when necessary, especially in complex or sensitive situations. For example, humans must ensure that the data used to train AI is accurate, diverse, and free from bias. Additionally, human oversight is important to prevent AI from making unethical decisions or mistakes, such as discriminatory hiring practices or flawed medical diagnoses. This ensures that AI continues to improve while adhering to ethical standards.
Conclusion
In this blog, we explored how AI learns through different methods like supervised, unsupervised, and reinforcement learning. Over time, AI improves by processing more data and adapting to new situations, which enhances its decision-making and accuracy. While AI can learn on its own, it still requires human oversight to ensure its learning is ethical and accurate. As AI continues to evolve, it’s transforming industries and improving technology, and it’s exciting to see how it will shape the future. Explore this growing field to see how AI can impact the world around you.
AI learns by processing data and identifying patterns on its own using algorithms, without needing direct human intervention for each task.
AI doesn’t learn like humans; it relies on data and algorithms to recognize patterns, while humans learn through experience and intuition.
AI needs large amounts of data to identify patterns and improve accuracy. More data helps AI make better predictions and decisions.